According to research firm IDC, “Decision intelligence is the missing piece of an organization’s technology stack, giving users the insights they need to make the best decisions with the best possible outcomes. This technology will change how information is synthesized, insights are developed, and decisions are made at scale.” Link
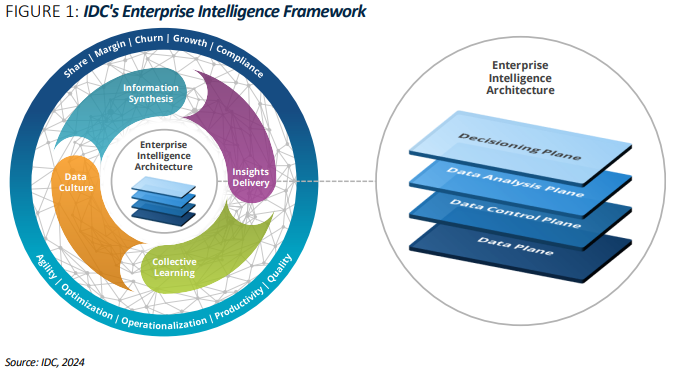
You must be logged in to post a comment.